Demystifying Agentic AI for Complex Service
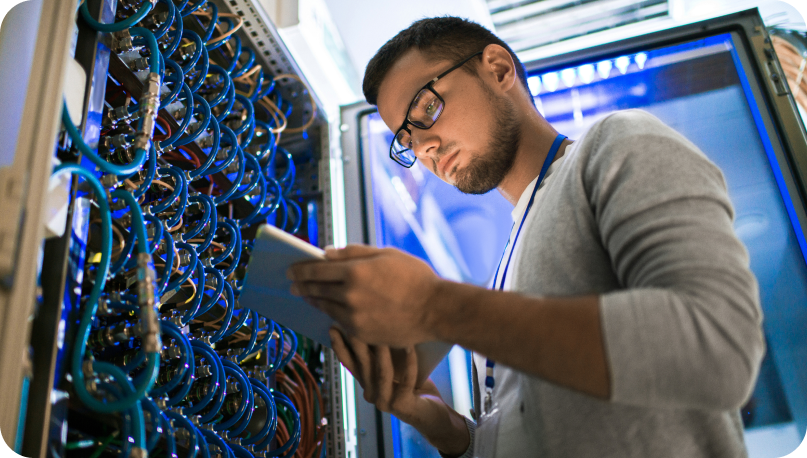
The world of generative AI for complex service is rapidly evolving, and with it comes an explosion of new terms and solutions that promise to transform how organizations operate. For global service leaders challenged with running and optimizing a service organization at scale with 100’s of people and 1000’s of products, just trying to educate yourself on “what’s in it for me” can feel overwhelming. With major vendors like Salesforce’s Agentforce, ServiceNow Now Assist, Microsoft Copilot, and emerging players like Sierra AI entering the market, it’s hard to distinguish hype from real, actionable solutions.
At the same time, service-specific solutions like Neuron7 are quietly revolutionizing how businesses address complex service needs with a focus on agentic AI issue resolution — AI that doesn’t just assist but acts with a degree of autonomy, and seamlessly partners with your skilled workforce to drive resolutions at scale.
In this blog post, we’ll demystify key concepts around agentic AI, discuss how service-specific solutions approach these challenges, and provide actionable insights for organizations looking to adopt AI in their service environments.
What is an Agent?
AI agents, or systems based on agentic principles, are capable of autonomous decision-making, adaptive behavior, and taking actions based on environmental inputs and internal goals. These systems process unstructured data (such as case subject, case description, logs, and telemetry data) and structured data (like error codes or model numbers) to generate insights. Based on the analysis of these inputs, the system can provide outputs such as troubleshooting steps, recommended knowledge base articles (KBs), manuals, SOPs, or even take actions on connected assets (e.g., performing remote diagnostics or triggering automated fixes). These outputs help achieve specific objectives, all without requiring human intervention at every decision point.
Key characteristics of agentic systems include:
- Autonomy: The system can make decisions and take actions independently.
- Goal-Oriented Behavior: The system operates to achieve specific goals.
- Perception: The system perceives input from the environment (data or user queries).
- Decision-Making: The system makes decisions based on perceived data.
- Action: The system performs actions based on its decisions.
- Adaptability: The system learns and adapts to new inputs over time.
How Is It Different from Generative AI?
Generative AI (e.g., OpenAI’s GPT or Claude by Anthropic) is designed to produce content—text, images, or code—based on a given prompt. While it’s effective for general-purpose tasks like drafting emails or generating customer responses, it lacks the specialized capabilities to:
- Understand the nuances of technical service data, like the steps that were taken to resolve an issue the last ten times it occurred.
- Navigate real-time problem-solving scenarios, most commonly when under a stressful situation like an outage.
- Integrate seamlessly into existing service workflows so your service team can easily work with agents without swivel-chairing to different platforms or screens.
Agentic AI issue resolution bridges this gap by acting with purpose and context, making it indispensable to global service teams.
The Pitfalls of Generic AI in Complex Service Environments

Many organizations have considered or deployed AI solutions like chatbots or large language models (LLMs) to enhance their service operations. While these tools offer some benefits, they often fail to address the unique needs of complex service environments.
Challenges with Generic AI:
- Contextual Understanding: Generic AI often struggles with domain-specific terminology or intricate troubleshooting workflows.
- Scalability: Generic solutions require extensive customization to scale across global teams and multilingual environments.
- Accuracy: LLMs are prone to “hallucinations”—providing incorrect or misleading information that can exacerbate service delays.
- Lack of Internal Expertise: Most service leaders we talk to lack the internal capacity and subject-matter expertise required to tune and refine models or prompts that Generic AI solutions require,
In contrast, solutions like Neuron7.ai are purpose-built to handle these complexities, using an AI-as-a-Service model to curate the best AI infrastructure and models per use-case or scenario, ensuring higher accuracy, faster resolution times, and seamless collaboration across geographically and culturally dispersed teams.
Is Neuron7 an Agentic Platform?
Absolutely. Neuron7 is more than just a search engine; it is an agentic AI issue resolution system that learns, adapts, and acts, transforming how enterprises search, discover, and act on information.
Using the characteristics outlined above Neuron7 is developed with goals to help deliver resolution accuracy at 90% and higher, has autonomy to process user queries, identify the key information to retrieve, add additional context, and provide actionable results without human intervention. It the uses advanced AI models (like NLP and entity recognition) to interpret user intent and context to deliver highly relevant resolution pathways. Neuron7 takes action to guide users by suggesting next steps or the next likely error, and continuously learns and adapts to user feedback, refining its decision making and improving performance over time.
So great, but what does this all mean for you?
Key Questions for Service Leaders
Before jumping into the AI race, service leaders should ask themselves:
- What are the specific pain points in our service operations? Start with the end-result and work backward to ensure the most pressing challenges for your service org are being addressed. Identifying KPIs will help determine where in the service continuum you’ll reap the most benefits.
- Do I have a seat at the table for org-wide AI initiatives? No doubt, there’s a team or committee looking at the best AI solutions to solve the widest range of use-cases from service, sales, HR and other departments. Have you built a strong POV on your needs? Have you articulated that across the organization? If you don't, someone else will.
- What’s the ROI we’re aiming for, and have we accurately accounted for the full Total Cost of Ownership (TCO)? Launching an AI tool requires more than models, software and data storage. You would need to hire or recruit subject-matter expertise, not only in AI but ones who understand the complexity of your business.
- Am I setting the right expectations with my team about the level of effort required? What realistic results can you achieve from implementing AI, how quickly can they be achieved, and what elements create the minimally necessary foundation to get started?
Putting it all Together
The world of service isn’t getting any simpler. With the increasing complexity of connected devices, the continued loss of knowledge due to employee churn and retirements, and the proliferation of data across disparate systems, service leaders need to stay on top of technology and how agentic AI can help them deliver exceptional customer service aligned with revenue and profit goals.
The future of service exists in systems that don’t just support decision making like analytics but drive it forward with transformative actions that change how teams discover, decision and deliver outcomes to customers.
If you’re unsure about how to get started we’re happy to help. Visit our AI Strategy Resource Center and book a consulting call with one of our experts today.
Further Reading and Resources
- For a technical perspective on Agentic AI in Neuron7, read the whitepaper here
- Learn more about Neuron7’s partnership with Salesforce here
- Visit us on the ServiceNow Store, or the Salesfore AppExchange