AI for Service: Build or Buy?
As AI initiatives become higher priorities and technologies become more accessible, many enterprise organizations consider building solutions in-house. A variety of questions typically arise when exploring the idea:
- Will general LLMs work for our needs?
- Can we just apply GPT?
- Is GenAI the answer for us?
- Can we just use RAG architecture or Vector Embedding Databases?
- Are there some use cases that make sense to buy vs. build?
- Do we have the right expertise?
For service organizations, the build approach is often the best path for simple use cases that require a bit of customization, while complex use cases in service environments with complex products find a faster path to success with the right AI solution partner.
The Challenges of Building
Service environments with complex products and thousands of potential issues require exceptional levels of accuracy for an AI solution to be effective. As a result, AI tools built on generally available LLMs and technologies fall far short of that threshold.
A couple of reasons why:
- General LLMs are trained on publicly available data and do not have the context and governance for accurate predictions.
- GenAI and GPT models represent an important piece of an advanced AI technology mix but are only as good the data they have access to and should not be viewed as a magic wand.
- Retrieval Augmented Generation (RAG) architecture and Vector Embedding Databases are great tools for identifying similar data, but can generate hallucinations (confident but wrong answers) when relied on alone to optimize LLM outputs. Service requires precision in understanding nuanced data. For example, in service “error 221” may be numerically similar to “error 222” but may not be related to the actual product or issue in any way.
These factors often combine to increase all-in costs well beyond initial expectations.
Accuracy Requires Time and Resources

For complex service environments, the power of fast, accurate AI is unlocked through a mix of technologies, including deep domain-specific LLMs with years of dedicated model training, and AI experts assigned to maintaining your accuracy.
In order to build in-house, an enterprise organization needs data scientists, which is one of the most competitive skill sets to hire. If you fully staff an in-house team, there can be further delays:
- Internal competition for data scientists may mean that your project gets bumped when another department’s AI initiative is prioritized
- If your project is prioritized, data scientists will need to spend significant time understanding your business, the complexity of the service challenges you’re trying to solve, and create a solution (which typically takes 36 months)
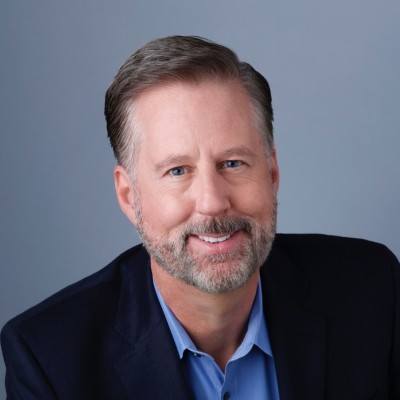
Get to 90% Accuracy Faster
With Service Resolution Intelligence, Neuron7 customers get to 90%+ accuracy faster with turn-by-turn guidance that learns from data and people.Built specifically for complex service environments, Neuron7 delivers AI solutions that are ready to go, ready to solve top use cases, and already in production and driving results at some of the world’s largest companies.
For more context about the foundational work that makes Neuron7 ready to take on your toughest service challenges, check out 7 Reasons Why Domain-Specific AI is Important.
If you want to jump start your AI strategy, get in touch. We’ll help you identify solutions and see AI in action in your service environment in weeks, not months or years.
Contact Us
Resolve issues faster with AI that understands your service data and continually learns as you use it.